Unlocking the Future: How Machine Learning is Transforming Industries
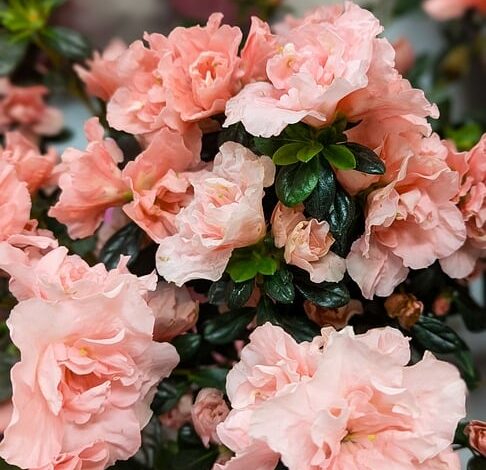
In today’s rapidly evolving technological landscape, machine learning (ML) stands out as a transformative force with the potential to revolutionize various industries. From healthcare to finance, and manufacturing to retail, the adoption of ML technologies is enabling organizations not only to enhance operational efficiencies but also to innovate and create new business models. As we explore the impact of machine learning, it’s crucial to understand how this technology is reshaping industries and unlocking future possibilities.
Understanding Machine Learning
Machine learning, a subset of artificial intelligence (AI), focuses on the development of algorithms that enable computers to learn from and make predictions based on data. Rather than relying on explicit programming, ML systems improve their performance as they are exposed to more data. This ability to learn from patterns in data has catalyzed a spectrum of applications that touch various aspects of business and everyday life.
Transformations Across Industries
1. Healthcare: Enhancing Patient Outcomes
In the healthcare sector, machine learning is spearheading advancements that are improving patient care and operational efficiency. Algorithms analyze vast datasets from electronic health records, enabling predictive analytics that can detect diseases early, suggest personalized treatment plans, and even predict patient outcomes.
For instance, ML models have shown remarkable success in interpreting medical images, such as X-rays and MRIs, with accuracy that often matches or surpasses that of trained radiologists. Furthermore, ML-driven clinical decision support systems assist healthcare providers by offering evidence-based recommendations tailored to individual patient profiles.
2. Finance: Risk Management and Fraud Detection
Machine learning is proving indispensable in the finance industry, particularly in areas like risk management, credit scoring, and fraud detection. Financial institutions apply ML algorithms to analyze transaction patterns, which helps in identifying suspicious activities in real-time, thereby mitigating fraud risks.
Moreover, through advanced credit scoring models that assess a more comprehensive range of consumer behaviors, lenders can make more informed decisions when extending credit. This nuanced approach allows for greater financial inclusion for individuals who may be overlooked by traditional assessment methods.
3. Manufacturing: Streamlining Operations
In manufacturing, machine learning is facilitating the adoption of smart factories, where data-driven decisions lead to optimized production processes. Predictive maintenance is one of the key applications; ML algorithms analyze equipment data to predict failures before they occur, reducing downtime and costs.
Additionally, ML is employed in supply chain management, where it helps optimize inventory levels, forecast demand, and streamline logistics. These advancements contribute not only to cost savings but also to a more responsive manufacturing ecosystem.
4. Retail: Personalization and Customer Experience
The retail industry is leveraging machine learning to enhance customer experience and drive sales. By analyzing shopping behavior and preferences, retailers can create personalized product recommendations, which not only improve customer satisfaction but also increase sales conversion rates.
Moreover, ML tools are used for inventory management, helping retailers predict customer demand with greater accuracy and manage stock levels effectively. The integration of ML-driven chatbots also enhances customer service, offering instant responses and personalized assistance to shoppers.
5. Transportation: Autonomous Vehicles and Route Optimization
The transportation sector is undergoing a transformation, heavily influenced by machine learning. Autonomous vehicles utilize ML algorithms to navigate and make real-time decisions on the road, significantly advancing safety and efficiency in transportation systems.
Furthermore, ride-sharing platforms leverage machine learning to optimize routes, predict demand, and enhance driver-partner matching. This not only improves service delivery but also contributes to reduced operational costs.
Ethical Considerations and Future Challenges
While the potential of machine learning is immense, it also comes with challenges and ethical considerations. Issues related to data privacy, algorithmic bias, and job displacement require careful navigation. The efficacy of ML algorithms often depends on the quality of data they are trained on, raising concerns about fairness and transparency.
As industries continue to integrate machine learning into their operations, it is crucial for stakeholders to prioritize ethical considerations, ensuring that technology serves the greater good and benefits all members of society.
Conclusion
Machine learning is undeniably unlocking the future of various industries, driving innovation and improving operational efficiencies across sectors. As organizations embrace this transformative technology, the potential benefits are vast, promising a future where businesses are not only smarter and more efficient but also more socially responsible. Continued investment in ML research and development, coupled with a commitment to ethical standards, will ensure that this remarkable technology fosters inclusive growth and progress in the years to come.